Deep Semantic Statistics Matching (D2SM) Denoising Network ECCV 2022, A New General Plug-and-play Component For Denoising
-
Kangfu Mei
JHU -
Vishal M. Patel
JHU -
Rui Huang
CUHK(SZ)
Abstract
The ultimate aim of image restoration like denoising is to find an exact correlation between the noisy and clear image domains. But the optimization of end-to-end denoising learning like pixel-wise losses is performed in a sample-to-sample manner, which ignores the intrinsic correlation of images, especially semantics. In this paper, we introduce the Deep Semantic Statistics Matching (D2SM) Denoising Network. It exploits semantic features of pretrained classification networks, then it implicitly matches the probabilistic distribution of clear images at the semantic feature space. By learning to preserve the semantic distribution of denoised images, we empirically find our method significantly improves the denoising capabilities of networks, and the denoised results can be better understood by high-level vision tasks. Comprehensive experiments conducted on the noisy Cityscapes dataset demonstrate the superiority of our method on both the denoising performance and semantic segmentation accuracy. Moreover, the performance improvement observed on our extended tasks including super-resolution and dehazing experiments shows its potentiality as a new general plug-and-play component.
What it is doing?
Preserving the semantics of denoised images. By exploiting t-SNE to reduce dimensions of semantic features and project them into 2D coordinates, we visualize the distributions of denoised animal images in the semantic feature space. Ours preserves most semantics as the clear images.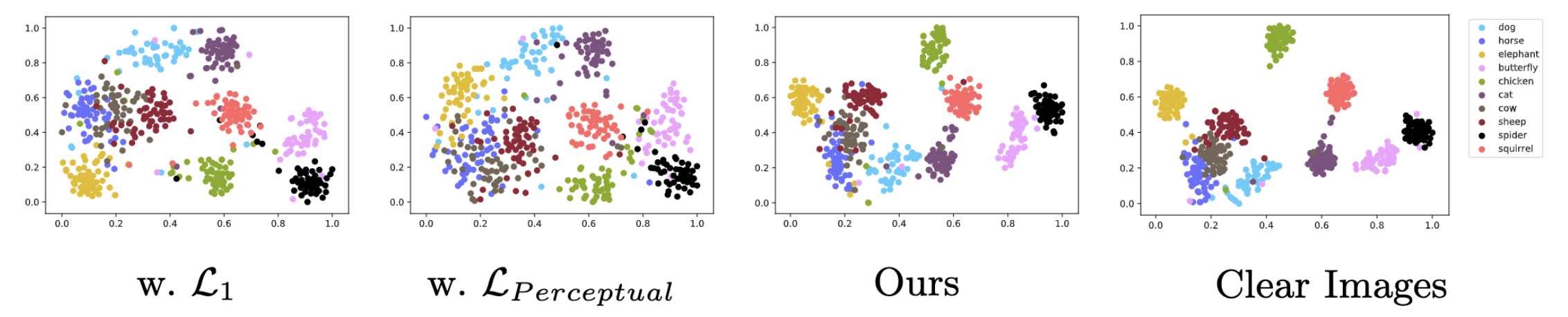
Citation
Acknowledgements
The website template was borrowed from Mip-NeRF 360.